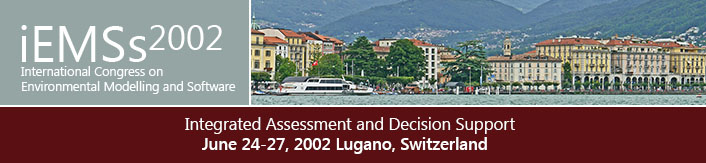
1st International Congress on Environmental Modelling and Software - Lugano, Switzerland - June 2002
Keywords
short-term wind speed prediction, neural network, time series, weather forecasting, environmental contamination
Start Date
1-7-2002 12:00 AM
Abstract
Predicting short-term wind speed is essential in order to model a system of prevention of environmental contamination produced by the effects of strong winds acting on goods (mainly crushed coal) discharged at a dock. The wind speed in a near future depends on the values of other meteorological variables in previous times. The values are obtained from a meteorological station with several sensors: wind speed, temperature, humidity, pressure ... We have used the SNNS simulator to obtain a neural network able to predict the wind speed 20 min. in advance, with the minimum possible error. The network inputs are basically historical values of the predicted variable as well as a number of other support variables. A feed-forward model has been elected with the aim of carrying out the treatment of the data. The algorithm used for the training phase has been back-propagation.
Local Short-Term Prediction of Wind Speed: A Neural Network Analysis
Predicting short-term wind speed is essential in order to model a system of prevention of environmental contamination produced by the effects of strong winds acting on goods (mainly crushed coal) discharged at a dock. The wind speed in a near future depends on the values of other meteorological variables in previous times. The values are obtained from a meteorological station with several sensors: wind speed, temperature, humidity, pressure ... We have used the SNNS simulator to obtain a neural network able to predict the wind speed 20 min. in advance, with the minimum possible error. The network inputs are basically historical values of the predicted variable as well as a number of other support variables. A feed-forward model has been elected with the aim of carrying out the treatment of the data. The algorithm used for the training phase has been back-propagation.