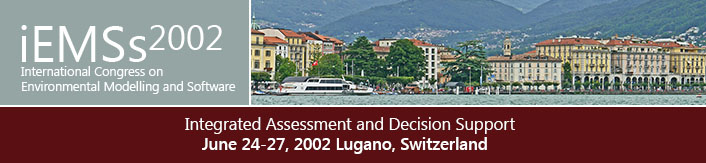
1st International Congress on Environmental Modelling and Software - Lugano, Switzerland - June 2002
Keywords
neural network, perceptron multilayer, hydrological scenario generation, multivariate timeseries analysis, water resources systems management
Start Date
1-7-2002 12:00 AM
Abstract
A multivariate non-linear model for synthetic generation of monthly discharge series is presented. The time series generator is built upon a multilayer feedforward neural network with an added multivariate random component normally distributed. The usual error backpropagation algorithm is used to train the network, with a sequential training scheme using shuffled patterns for a stochastic search in the weight space. The proposed model generates inputs to a wider methodology developed for simulation of the probabilistic managing of the upper Tagus River system in Spain. The model is validated in terms of its ability to reproduce some relevant statistics directly related to important features from the water resources system managing point of view, including droughts and storage derived statistics. The generator was inserted into a decision support system, and a variety of possible future hydrological scenarios in the river system were simulated, with particular consideration of demand failures probabilities under different assumptions and previous hydrological states of the system. For comparison purposes, similar experiments were also undertaken using synthetic series generated with a second-order autoregressive multivariate model, AR(2). The results obtained show higher percentages of demand failures when inputs from the artificial neural network generator (ANN) are used. The case study illustrates another practical application of ANN approaches, adequately combined with other frequently used tools in the context of water resources systems planning and management.
An Artificial Neural Networks Modeling Approach Applied to the Probabilistic Management of Water Resources Systems
A multivariate non-linear model for synthetic generation of monthly discharge series is presented. The time series generator is built upon a multilayer feedforward neural network with an added multivariate random component normally distributed. The usual error backpropagation algorithm is used to train the network, with a sequential training scheme using shuffled patterns for a stochastic search in the weight space. The proposed model generates inputs to a wider methodology developed for simulation of the probabilistic managing of the upper Tagus River system in Spain. The model is validated in terms of its ability to reproduce some relevant statistics directly related to important features from the water resources system managing point of view, including droughts and storage derived statistics. The generator was inserted into a decision support system, and a variety of possible future hydrological scenarios in the river system were simulated, with particular consideration of demand failures probabilities under different assumptions and previous hydrological states of the system. For comparison purposes, similar experiments were also undertaken using synthetic series generated with a second-order autoregressive multivariate model, AR(2). The results obtained show higher percentages of demand failures when inputs from the artificial neural network generator (ANN) are used. The case study illustrates another practical application of ANN approaches, adequately combined with other frequently used tools in the context of water resources systems planning and management.