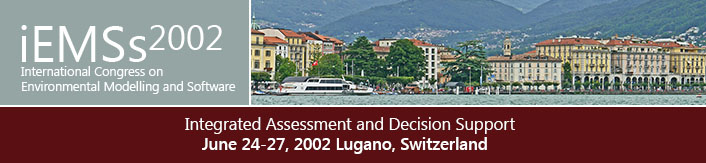
1st International Congress on Environmental Modelling and Software - Lugano, Switzerland - June 2002
Keywords
artificial neural networks, inverse problem, groundwater, uncertainty, parameter
Start Date
1-7-2002 12:00 AM
Abstract
In this paper, prediction capability of a hybrid Artificial Neural Networks (ANN) was investigatedto solve the groundwater inverse problem. Initially, a Multi Layer Perceptron (MLP) network was developedand it was found that network produced better results when the target range of the parameters is smaller.Therefore, a Self-Organising Network (SON) was used to identify the objective subrange of the parameterand then the MLP model was employed to obtain final estimates. The data for the ANN was obtained from anumerical model that was utilised to simulate the solute transport in saturated groundwater flow. The forwardproblem of the numerical model was solved to generate solute concentration data for range of parameters.Those input data was fed into a MLP ANN to train the network along with corresponding parameter values.Sufficiently trained ANN model was used to estimate hydraulic conductivity (single parameter), andhydraulic conductivity and longitudinal dispersion coefficient (two parameters). First, the approach wastested on synthetic data to identify its feasibility and robustness. Then an experimental dataset that wasobtained from an artificial aquifer was used to validate the method. It was found that ANNs produce accurateestimates in the presence of uncertainty. However, ANN are able to produce accurate results only if thepattern of the dataset that use to estimate parameters are similar to that of the training data. Therefore, it isimportant to adequately simulate the aquifer system in question by a large enough training dataset. However,due to the stochastic nature of the real world heterogeneous aquifers, it is not a trivial undertaking to identifythe behaviour of the aquifer. Furthermore, as ANN’s extrapolation capabilities beyond its calibration range isnot reliable, it is necessary to set a calibration range sufficient to meet the limits of actual data. Therefore,prior information of the system is of utmost importance to obtain reasonably accurate estimates.
Solving the Inverse Problem in Stochastic Groundwater Modelling with Artificial Neural Networks
In this paper, prediction capability of a hybrid Artificial Neural Networks (ANN) was investigatedto solve the groundwater inverse problem. Initially, a Multi Layer Perceptron (MLP) network was developedand it was found that network produced better results when the target range of the parameters is smaller.Therefore, a Self-Organising Network (SON) was used to identify the objective subrange of the parameterand then the MLP model was employed to obtain final estimates. The data for the ANN was obtained from anumerical model that was utilised to simulate the solute transport in saturated groundwater flow. The forwardproblem of the numerical model was solved to generate solute concentration data for range of parameters.Those input data was fed into a MLP ANN to train the network along with corresponding parameter values.Sufficiently trained ANN model was used to estimate hydraulic conductivity (single parameter), andhydraulic conductivity and longitudinal dispersion coefficient (two parameters). First, the approach wastested on synthetic data to identify its feasibility and robustness. Then an experimental dataset that wasobtained from an artificial aquifer was used to validate the method. It was found that ANNs produce accurateestimates in the presence of uncertainty. However, ANN are able to produce accurate results only if thepattern of the dataset that use to estimate parameters are similar to that of the training data. Therefore, it isimportant to adequately simulate the aquifer system in question by a large enough training dataset. However,due to the stochastic nature of the real world heterogeneous aquifers, it is not a trivial undertaking to identifythe behaviour of the aquifer. Furthermore, as ANN’s extrapolation capabilities beyond its calibration range isnot reliable, it is necessary to set a calibration range sufficient to meet the limits of actual data. Therefore,prior information of the system is of utmost importance to obtain reasonably accurate estimates.