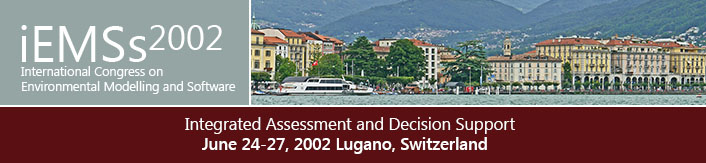
1st International Congress on Environmental Modelling and Software - Lugano, Switzerland - June 2002
Paper/Poster/Presentation Title
Linearisation of Highly Resolved Substance Transport Models by Temporal Aggregation of Model Outputs
Keywords
temporal aggregation, linearity, soil/soiln model, substance transport, drainage
Start Date
1-7-2002 12:00 AM
Abstract
Process-oriented models that involve strongly non-linear responses and are driven by highlyresolved meteorological inputs are often used to predict substance transport over fairly long periods of time.Therefore, it is of great interest to ascertain whether the non-linear structure is also essential for predictingtemporally aggregated model outputs. Here, ordinary least squares regression was employed to identify linearrelationships between model outputs and inputs expressed on different temporal scales. Examination of twosimple theoretical models showed that aggregation of outputs and inputs improved the linearity of models,provided the models included memory effects. This was confirmed in the analysis of the soil-water andnitrogen transport model SOIL/SOILN. Moreover, linear predictors that involved highly resolved inputsproved to be superior to linear predictors based on temporally aggregated inputs. The presence of linearstructures facilitates the extrapolation of point models to larger areas, because it allows the use of spatiallyaggregated inputs to calculate spatially aggregated outputs.
Linearisation of Highly Resolved Substance Transport Models by Temporal Aggregation of Model Outputs
Process-oriented models that involve strongly non-linear responses and are driven by highlyresolved meteorological inputs are often used to predict substance transport over fairly long periods of time.Therefore, it is of great interest to ascertain whether the non-linear structure is also essential for predictingtemporally aggregated model outputs. Here, ordinary least squares regression was employed to identify linearrelationships between model outputs and inputs expressed on different temporal scales. Examination of twosimple theoretical models showed that aggregation of outputs and inputs improved the linearity of models,provided the models included memory effects. This was confirmed in the analysis of the soil-water andnitrogen transport model SOIL/SOILN. Moreover, linear predictors that involved highly resolved inputsproved to be superior to linear predictors based on temporally aggregated inputs. The presence of linearstructures facilitates the extrapolation of point models to larger areas, because it allows the use of spatiallyaggregated inputs to calculate spatially aggregated outputs.