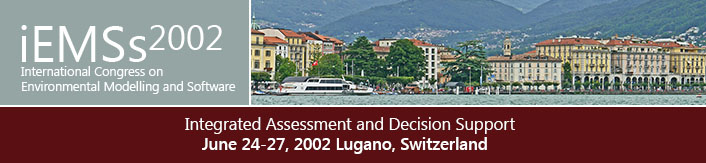
1st International Congress on Environmental Modelling and Software - Lugano, Switzerland - June 2002
Keywords
climate downscaling, daily precipitation, skill of predictors, artificial neural networks
Start Date
1-7-2002 12:00 AM
Abstract
The urgent need for realistic regional climate change scenarios has led to a plethora of empirical downscaling techniques. In many cases widely differing predictors are used, making comparative evaluation difficult. Additionally, it is not clear that the chosen predictors are always the most important. These limitations and the lack of physics in empirical downscaling highlight the need for a systematic assessment of the performance of physically meaningful predictors and their relevance in surface climate parameters. Accordingly, the objectives of this study are twofold: To examine the skill and errors of 29 individual atmospheric predictors of area-averaged daily precipitation in 15 locations that encompass a wide variety of climate regimes, and to determine the best combination of these to empirically model daily precipitation during the winter and summer seasons. The atmospheric predictors utilized in this study are from the National Center for Environmental Prediction (NCEP) Reanalysis. This work is not concerned with evaluating a particular downscaling methodology, but rather with evaluating the relative skill of physically meaningful predictors that are able to capture different sources of variation. The results indicate that humidity and geopotential heights at mid-tropospheric levels are the two most relevant controls of daily precipitation in all the locations and seasons analyzed. A less ubiquitous role is played by the tropospheric thickness, and the surface meridional and 850-hPa wind components, which appear to be regionally and seasonally dependent. Poor skill is found in the near-equatorial regions and in the Tropics where convective processes dominate and, possibly, where the reanalysis data sets utilized are most deficient. The warm season hemisphere is characterized by the largest errors, likely also due to the enhanced role of convection and sub-grid scale processes during this season. Discrepancies between the performance of the downscaling at grid cell (2o lat x 2.5o lon) and local scales further indicate the sensitivity to the spatial resolution of the predictors.
Relative Performance of Empirical Predictors of Daily Precipitation
The urgent need for realistic regional climate change scenarios has led to a plethora of empirical downscaling techniques. In many cases widely differing predictors are used, making comparative evaluation difficult. Additionally, it is not clear that the chosen predictors are always the most important. These limitations and the lack of physics in empirical downscaling highlight the need for a systematic assessment of the performance of physically meaningful predictors and their relevance in surface climate parameters. Accordingly, the objectives of this study are twofold: To examine the skill and errors of 29 individual atmospheric predictors of area-averaged daily precipitation in 15 locations that encompass a wide variety of climate regimes, and to determine the best combination of these to empirically model daily precipitation during the winter and summer seasons. The atmospheric predictors utilized in this study are from the National Center for Environmental Prediction (NCEP) Reanalysis. This work is not concerned with evaluating a particular downscaling methodology, but rather with evaluating the relative skill of physically meaningful predictors that are able to capture different sources of variation. The results indicate that humidity and geopotential heights at mid-tropospheric levels are the two most relevant controls of daily precipitation in all the locations and seasons analyzed. A less ubiquitous role is played by the tropospheric thickness, and the surface meridional and 850-hPa wind components, which appear to be regionally and seasonally dependent. Poor skill is found in the near-equatorial regions and in the Tropics where convective processes dominate and, possibly, where the reanalysis data sets utilized are most deficient. The warm season hemisphere is characterized by the largest errors, likely also due to the enhanced role of convection and sub-grid scale processes during this season. Discrepancies between the performance of the downscaling at grid cell (2o lat x 2.5o lon) and local scales further indicate the sensitivity to the spatial resolution of the predictors.