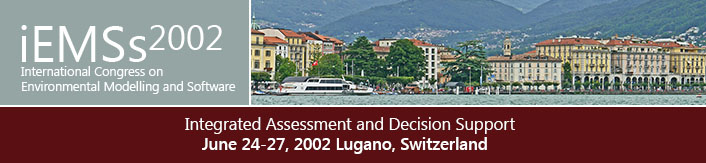
1st International Congress on Environmental Modelling and Software - Lugano, Switzerland - June 2002
Paper/Poster/Presentation Title
A neural emission-receptor model for ozone reduction planning
Keywords
neural network, ozone ground pollution, lombardy region
Start Date
1-7-2002 12:00 AM
Abstract
Ground level ozone pollution is a complex phenomenon heavily affecting industrialized and populated areas. Ozone is produced by a series of photochemical reactions, activated by the emissions of nitrogen oxides and volatile organic compounds and may reach maximum concentrations at kilometers of distance form the precursors sources, depending on the meteorological conditions. Models to compute ozone concentrations are equally complex and cannot be directly used to optimize emission reduction policies. For this reason, a neural network has been trained on the results of a photochemical model (CALGRID) to represent the emission-receptor relationships in critical conditions. Such a network in then entered in an optimization problem that determines the least cost alternatives to obtain a given air quality standard. The decision variables of the problem are the emission reductions of ozone precursors in each industrial sector. These reductions are in turn the result of the application of a number of technologies, whose costs and performances are known. The approach has been used to estimate the optimal reduction alternatives for a region in Northern Italy and showed that a consistent improvement of air quality can be attained with moderate investments, provided that these are concentrated in some sectors, such as road transport and industrial solvents, that have a major impact on ozone dynamics.
A neural emission-receptor model for ozone reduction planning
Ground level ozone pollution is a complex phenomenon heavily affecting industrialized and populated areas. Ozone is produced by a series of photochemical reactions, activated by the emissions of nitrogen oxides and volatile organic compounds and may reach maximum concentrations at kilometers of distance form the precursors sources, depending on the meteorological conditions. Models to compute ozone concentrations are equally complex and cannot be directly used to optimize emission reduction policies. For this reason, a neural network has been trained on the results of a photochemical model (CALGRID) to represent the emission-receptor relationships in critical conditions. Such a network in then entered in an optimization problem that determines the least cost alternatives to obtain a given air quality standard. The decision variables of the problem are the emission reductions of ozone precursors in each industrial sector. These reductions are in turn the result of the application of a number of technologies, whose costs and performances are known. The approach has been used to estimate the optimal reduction alternatives for a region in Northern Italy and showed that a consistent improvement of air quality can be attained with moderate investments, provided that these are concentrated in some sectors, such as road transport and industrial solvents, that have a major impact on ozone dynamics.