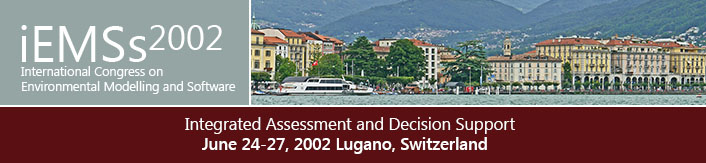
1st International Congress on Environmental Modelling and Software - Lugano, Switzerland - June 2002
Keywords
statistical learning theory, mechanistic modelling, uncertainty models, model performance, exact and empirical measures of model performance
Start Date
1-7-2002 12:00 AM
Abstract
The traditional model validation procedure has been criticized in many areas of environmentalengineering. In this paper, it is suggested to replace it by a more reasonable procedure: “model evaluation”.A framework for model evaluation and structure selection (FMESS) is presented. Based on statisticallearning theory, this framework considers the model identification step as a learning problem. The modelevaluation process is based on one single criterion: model performance. The latter is measured by amathematical deviation between the model prediction and reality. Although it is an exact measure of modelperformance, this deviation cannot be computed, but can be related to the empirical measure that systemmodellers have traditionally used in the steps of model identification and validation. The relationshipbetween the exact and empirical measures is called an uncertainty model. Two uncertainty models arepresented in this paper. For these models to be valid, a set of conditions with regard to the system uncertaintyneeds to be satisfied. Although quite weak, these conditions may not always hold true in the case of complexenvironmental systems. Mechanistic information on the system’s dynamic processes would be required toensure the fulfilment of these conditions.
Integrating Mechanistic Modelling and Statistical Learning Theoretic-Methods: initial steps toward a strategy for model evaluation and structure selection
The traditional model validation procedure has been criticized in many areas of environmentalengineering. In this paper, it is suggested to replace it by a more reasonable procedure: “model evaluation”.A framework for model evaluation and structure selection (FMESS) is presented. Based on statisticallearning theory, this framework considers the model identification step as a learning problem. The modelevaluation process is based on one single criterion: model performance. The latter is measured by amathematical deviation between the model prediction and reality. Although it is an exact measure of modelperformance, this deviation cannot be computed, but can be related to the empirical measure that systemmodellers have traditionally used in the steps of model identification and validation. The relationshipbetween the exact and empirical measures is called an uncertainty model. Two uncertainty models arepresented in this paper. For these models to be valid, a set of conditions with regard to the system uncertaintyneeds to be satisfied. Although quite weak, these conditions may not always hold true in the case of complexenvironmental systems. Mechanistic information on the system’s dynamic processes would be required toensure the fulfilment of these conditions.