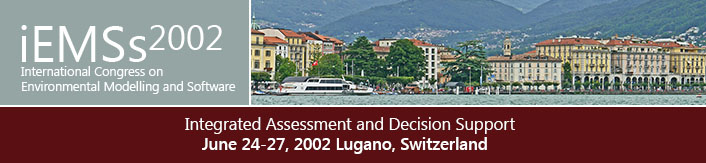
1st International Congress on Environmental Modelling and Software - Lugano, Switzerland - June 2002
Paper/Poster/Presentation Title
Keywords
credal classification, imprecise probabilities, naive credal classifier, imprecise dirichlet model, agricultural data
Start Date
1-7-2002 12:00 AM
Abstract
Classifiers that aim at doing reliable predictions should rely on carefully elicited prior knowledge. Often this is not available so they should be able to start learning from data in condition of prior ignorance. This paper shows empirically, on an agricultural data set, that established methods of classification do not always adhere to this principle. Common ways to represent prior ignorance are shown to have an overwhelming weight compared to the information in the data, producing overconfident predictions. This point is crucial for problems, such as environmental ones, where prior knowledge is often scarce and even the data may not be known precisely. Credal classification, and in particular the naive credal classifier, are proposed as more faithful ways to represent states of ignorance. We show that with credal classification, conditions of ignorance may limit the power of the inferences, not the reliability of the predictions.
Credal Classification for Mining Environmental Data
Classifiers that aim at doing reliable predictions should rely on carefully elicited prior knowledge. Often this is not available so they should be able to start learning from data in condition of prior ignorance. This paper shows empirically, on an agricultural data set, that established methods of classification do not always adhere to this principle. Common ways to represent prior ignorance are shown to have an overwhelming weight compared to the information in the data, producing overconfident predictions. This point is crucial for problems, such as environmental ones, where prior knowledge is often scarce and even the data may not be known precisely. Credal classification, and in particular the naive credal classifier, are proposed as more faithful ways to represent states of ignorance. We show that with credal classification, conditions of ignorance may limit the power of the inferences, not the reliability of the predictions.