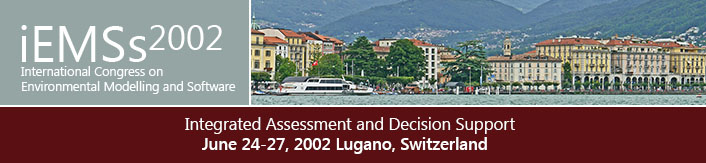
1st International Congress on Environmental Modelling and Software - Lugano, Switzerland - June 2002
Keywords
artificial neural networks, flood forecasting, conceptual model, black-box model
Start Date
1-7-2002 12:00 AM
Abstract
The paper presents a comparison of lumped runoff modelling approaches, aimed at the realtime forecasting of flood events, based on or integrating Artificial Neural Networks (ANNs). ANNs are used in two ways: (a) as black-box type runoff simulation models or (b) for the real-time improvement of the discharge forecasts issued by a conceptual-type rainfall-runoff model. As far as the coupling of ANNs with a conceptual model is concerned, feed forward neural networks are used as univariate time-series analysis techniques both for forecasting the future rainfall values to be provided as input to the hydrological model and for updating the river discharges issued by the model. A real-world case study is developed on the Sieve River basin (Central Italy) and future river flows are first predicted using artificial neural networks as blackbox models, both with the only use of past flow observations and with the addition of exogenous inputs, that is previous rainfall depths. It is then applied the conceptual model and it is assessed the improvement allowed when integrating it with the ANN rainfall prediction and output updating modules. The results show that the ANN black-box model with exogenous input, when trained on a adequately representative data set, gives the best forecasting performances over the validation set. On the other hand, if the training set does not cover all the variety of events present in the validation set, for example if the major events are subtracted, the flood features were found to be better captured by the conceptual model coupled with pre and postprocessing ANN modules, thus demonstrating a greater generalisation ability of such approach.
Flood Forecasting Using Artificial Neural Networks in Black-Box and Conceptual Rainfall-Runoff Modelling
The paper presents a comparison of lumped runoff modelling approaches, aimed at the realtime forecasting of flood events, based on or integrating Artificial Neural Networks (ANNs). ANNs are used in two ways: (a) as black-box type runoff simulation models or (b) for the real-time improvement of the discharge forecasts issued by a conceptual-type rainfall-runoff model. As far as the coupling of ANNs with a conceptual model is concerned, feed forward neural networks are used as univariate time-series analysis techniques both for forecasting the future rainfall values to be provided as input to the hydrological model and for updating the river discharges issued by the model. A real-world case study is developed on the Sieve River basin (Central Italy) and future river flows are first predicted using artificial neural networks as blackbox models, both with the only use of past flow observations and with the addition of exogenous inputs, that is previous rainfall depths. It is then applied the conceptual model and it is assessed the improvement allowed when integrating it with the ANN rainfall prediction and output updating modules. The results show that the ANN black-box model with exogenous input, when trained on a adequately representative data set, gives the best forecasting performances over the validation set. On the other hand, if the training set does not cover all the variety of events present in the validation set, for example if the major events are subtracted, the flood features were found to be better captured by the conceptual model coupled with pre and postprocessing ANN modules, thus demonstrating a greater generalisation ability of such approach.