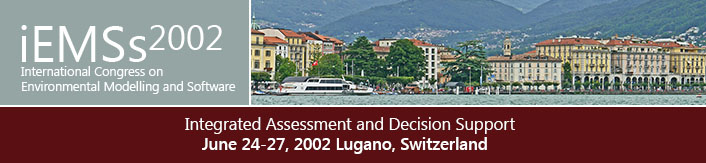
1st International Congress on Environmental Modelling and Software - Lugano, Switzerland - June 2002
Keywords
salinity intrusion, san francisco bay delta modeling, neural network, genetic algorithm optimization
Start Date
1-7-2002 12:00 AM
Abstract
Salinity intrusion is a significant issue in the San Francisco Bay Delta because this estuarycontributes substantially to fresh water supply for much of northern California. Traditionally, salinity alongthe estuary has been measured using monitoring instruments installed at various locations within theSacramento-San Joaquin River Delta system, and these measurements are used to predict future conditionsand evaluate the water management alternatives. The California Department of Water Resources DeltaSimulation Model (DWRDSM) is used for this type of analysis. DWRDSM is an unsteady one-dimensionalhydrodynamic and salt transport model. It can provide estimates of salinity at almost any location within theBay-Delta system. However, like many numerical models, when simulating the dynamics of a large complexsystem, the processing time can be extremely long, which makes it difficult to evaluate a large number ofmanagement scenarios in a short time. Statistically-based models of the flow and salinity have been triedand found lacking; classical time series analysis is often linear or requires transformation to a stationary timeseries that renders the resulting model unsuitable, since the salinity intrusion problem is a complex non-linearproblem. Consequently, there remains a need for a reliable and fast method to predict salinity intrusion inthe Sacramento-San Joaquin River Delta system. In this paper, such a method is advocated: GeneticAlgorithms (GA) are used to optimize a previously built Artificial Neural Network (ANN) that reliablypredicts periodic salinity intrusion in the San Francisco Bay Estuary. Results indicate that the GA providedan efficient method of optimizing the ANN model to predict salinity. The reliability of the ANN using theGA includes prediction of salinity to an accuracy of ±10% at hourly intervals for any time period in the year.
Optimization of a Model to Predict Salinity Intrusion in San Francisco Bay Estuary Using a Genetic Algorithm
Salinity intrusion is a significant issue in the San Francisco Bay Delta because this estuarycontributes substantially to fresh water supply for much of northern California. Traditionally, salinity alongthe estuary has been measured using monitoring instruments installed at various locations within theSacramento-San Joaquin River Delta system, and these measurements are used to predict future conditionsand evaluate the water management alternatives. The California Department of Water Resources DeltaSimulation Model (DWRDSM) is used for this type of analysis. DWRDSM is an unsteady one-dimensionalhydrodynamic and salt transport model. It can provide estimates of salinity at almost any location within theBay-Delta system. However, like many numerical models, when simulating the dynamics of a large complexsystem, the processing time can be extremely long, which makes it difficult to evaluate a large number ofmanagement scenarios in a short time. Statistically-based models of the flow and salinity have been triedand found lacking; classical time series analysis is often linear or requires transformation to a stationary timeseries that renders the resulting model unsuitable, since the salinity intrusion problem is a complex non-linearproblem. Consequently, there remains a need for a reliable and fast method to predict salinity intrusion inthe Sacramento-San Joaquin River Delta system. In this paper, such a method is advocated: GeneticAlgorithms (GA) are used to optimize a previously built Artificial Neural Network (ANN) that reliablypredicts periodic salinity intrusion in the San Francisco Bay Estuary. Results indicate that the GA providedan efficient method of optimizing the ANN model to predict salinity. The reliability of the ANN using theGA includes prediction of salinity to an accuracy of ±10% at hourly intervals for any time period in the year.