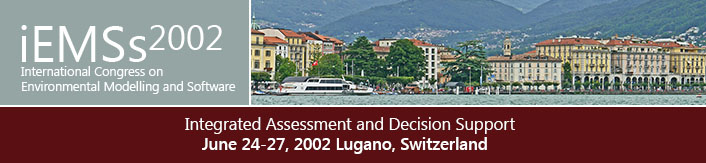
1st International Congress on Environmental Modelling and Software - Lugano, Switzerland - June 2002
Keywords
annual rainfall, hidden-state markov model, parameter uncertainty, bayesian
Start Date
1-7-2002 12:00 AM
Abstract
In the past, stochastic modelling approaches generally assumed no variation in the parameters between years. There is a growing awareness of long term persistence in climatic data in the form of wetter and drier years. To take this information into account, model parameters should be varied in some way. The hidden-state Markov (HSM) model assumes that the climate is composed of two states, either a dry state (low rainfall year) or a wet state (high rainfall year). This approach provides an explicit mechanism for the HSM model to simulate the influence of quasi-periodic phenomenon such as ENSO. Separate distributions are used to model the rainfall in the two states. The states are not known a priori and are estimated along with the model parameters. For model calibration, a Bayesian framework is used to infer the distribution of the model parameters. A Markov Chain Monte Carlo method known as Gibbs sampler is used to estimate the parameters and their uncertainties. The HSM model was applied to 44 rainfall stations located in various parts of Australia and the results indicated that 32 stations are either highly likely to or could possibly have two states. One thousand replicates each of length equal to the historical data were generated and several model evaluation statistics computed. The HSM model satisfactorily preserved all the model evaluation statistics.
Modelling Annual Rainfall Using a Hidden-State Markov Model
In the past, stochastic modelling approaches generally assumed no variation in the parameters between years. There is a growing awareness of long term persistence in climatic data in the form of wetter and drier years. To take this information into account, model parameters should be varied in some way. The hidden-state Markov (HSM) model assumes that the climate is composed of two states, either a dry state (low rainfall year) or a wet state (high rainfall year). This approach provides an explicit mechanism for the HSM model to simulate the influence of quasi-periodic phenomenon such as ENSO. Separate distributions are used to model the rainfall in the two states. The states are not known a priori and are estimated along with the model parameters. For model calibration, a Bayesian framework is used to infer the distribution of the model parameters. A Markov Chain Monte Carlo method known as Gibbs sampler is used to estimate the parameters and their uncertainties. The HSM model was applied to 44 rainfall stations located in various parts of Australia and the results indicated that 32 stations are either highly likely to or could possibly have two states. One thousand replicates each of length equal to the historical data were generated and several model evaluation statistics computed. The HSM model satisfactorily preserved all the model evaluation statistics.